Machine learning for material science photovoltaic

Machine learning–assisted molecular design and efficiency
Machine learning can be a powerful tool to prescreen new materials, thus accelerating the development of the OPV field. In the process of finding high-performance materials for organic photovoltaics (OPVs), it is meaningful if one can establish the relationship between chemical structures and photovoltaic properties even before synthesizing

Machine learning-assisted molecular design for high-performance
Wenbo Sun et al. Machine learning–assisted molecular design and efficiency prediction for high-performance organic photovoltaic materials, Science Advances (2019). DOI: 10.1126/sciadv.aay4275

Machine Learning for Organic Photovoltaic Polymers: A
ability. Machine learning works on the basis of statistical data fit. The change of volume of data significantly changes the performance of machine learning models. There are large number of machine learning models, the prediction ability also strongly depends on the used model. In material science especially, polymer solar cells, its use in

Comprehensive review of advances in machine-learning-driven
The photovoltaic properties of perovskite layers have been substantially improved over the past few years. These enhancements involve diverse strategies, such as altering the crystal structure through doping or substituting organic constituents, metal, and the halide with alternative counterparts [8].The extensive exploration of solvent combinations, anti-solvents, and

Machine Learning for Photovoltaic Applications
Machine learning ( ML) can be broadly described as a range of methods that have the capability to learn and improve without explicit programming, unlike rule-based computer programming (Samuel 1959). The algorithms use data to develop models that automatically identify correlations and patterns to address a problem.

Rapid Discovery of Ferroelectric Photovoltaic Perovskites and Material
DOI: 10.1002/SMTD.201900360 Corpus ID: 198394317; Rapid Discovery of Ferroelectric Photovoltaic Perovskites and Material Descriptors via Machine Learning @article{Lu2019RapidDO, title={Rapid Discovery of Ferroelectric Photovoltaic Perovskites and Material Descriptors via Machine Learning}, author={Shuaihua Lu and Qionghua Zhou and

Discovery of Novel Two-Dimensional Photovoltaic Materials
In this work, an efficient method is developed based on the machine learning (ML) algorithm combined with high-throughput screening that provides an efficient way of searching for novel 2DPV materials, but can be applied to a broad field of functional material exploring. Searching for novel two-dimensional photovoltaic (2DPV) materials with high performance is an important

Machine learning driven building integrated photovoltaic (BIPV
The building sector is responsible for a significant amount of global energy consumption and greenhouse gas emissions [1], [2].Fossil fuels continue to dominate the energy landscape, which has led to environmental and economic concerns [3] response to the urgent need to reduce this environmental impact, renewable energy solutions, such as photovoltaics (PV), have gained

Perovskite-Based Materials for Photovoltaic Applications: A Machine
2.1 Targeted Properties. There is a direct correlation between material properties and material applications. To successfully implement machine learning, various objectives must be clearly stated before the relevant steps can be undertaken, including the selection of the most appropriate machine learning techniques.
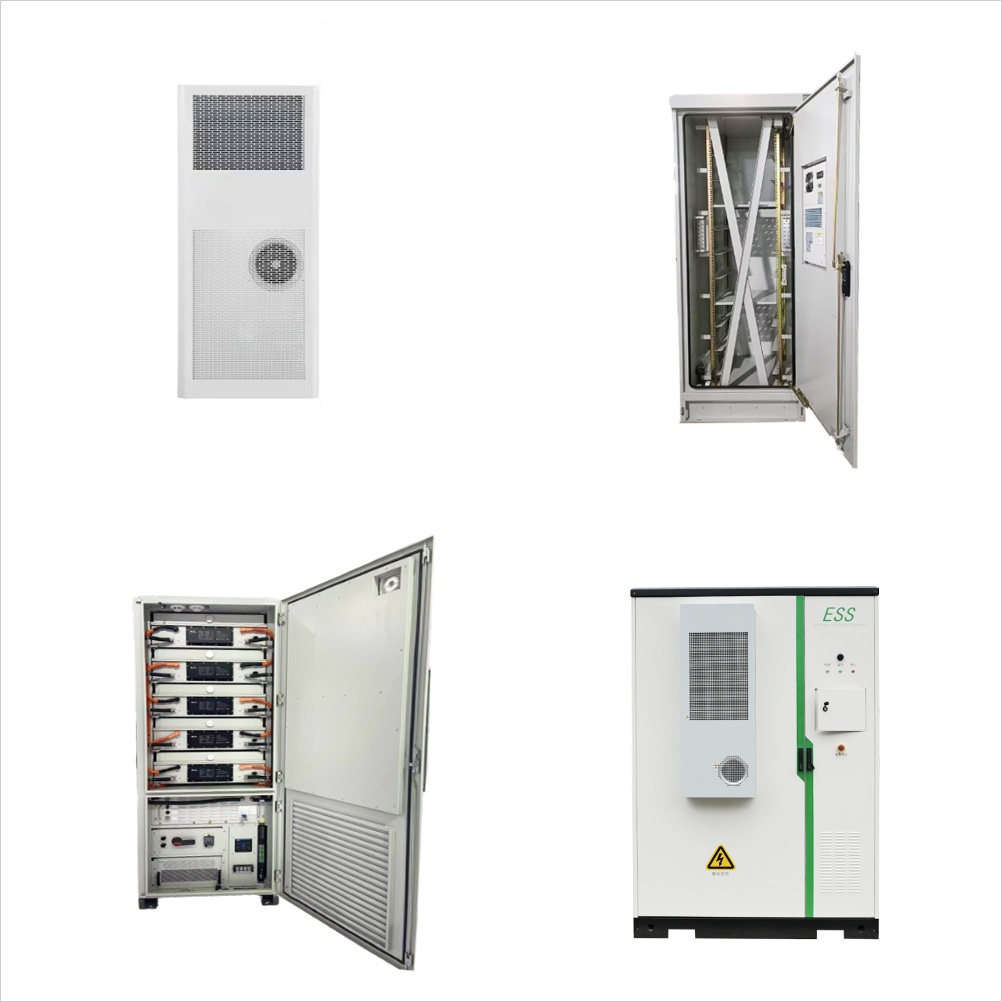
Using machine learning in photovoltaics to create smarter and
The wider application of machine learning to PV systems could therefore forge a Conventional technologies face several challenges and there has been remarkable progress made in the field of computer science and statistics. raising its temperature and enabling it to transfer heat to other spaces or materials [5]. Photovoltaic (PV

Predicting Inorganic Photovoltaic Materials with Efficiencies
The long research cycle and waste involved in traditional photovoltaic research can be alleviated significantly by novel material discovery technologies; density functional theory (DFT) high-throughput calculations 2, 3 and the machine learning (ML) 4, 5 method have emerged in recent years, which dramatically increase the accuracy and

Machine learning-driven design of promising perovskites for
In the future, ML will be more widely applied in materials science (e.g. PV materials) with the fast-growing development of computational methods and modern computer technologies. Big-data science in porous materials: materials genomics and machine learning. Chem. Rev., 120 (2020), pp. 8066-8129. Crossref View in Scopus Google Scholar [32

Editorial: Machine Learning in Materials Science
Editorial: Machine Learning in Materials Science Cite This: J. Chem. Inf. Model. 2024, 64, 3959−3960 Read Online ACCESS Metrics & More Article Recommendations I n the realm of materials science, where the exploration of new compounds and their properties can be painstakingly slow, artificialintelligence (AI), including machine learning

Material machine learning for alloys: Applications, challenges and
The coefficients of determinations were 0.99 and 0.96 for extreme learning machine and SVR, respectively. Extreme learning machine model provides wear loss prediction based on known and accessible parameters (sample production parameters, hardness, sliding distance, surface roughness) without long and expensive wear tests.

Enhancing solar photovoltaic energy production prediction using
Solar photovoltaic (PV) systems, integral for sustainable energy, face challenges in forecasting due to the unpredictable nature of environmental factors influencing energy output. This study

Machine Learning for Organic Photovoltaic Polymers: A
Machine learning is a powerful tool that can provide a way to revolutionize the material science. Its use for the designing and screening of materials for polymer solar cells is also increasing. Search of efficient polymeric materials for solar cells is really difficult task. Researchers have synthesized and fabricated so many materials. Sorting the results and get

Solar Energy Materials and Solar Cells
To address these challenges, the convergence of machine learning (ML) methodologies with materials science research marks a transformative shift in contemporary approaches to new energy material design and discovery [[8], [9], [10]]. Moreover, the application of ML has significantly contributed to unraveling the structure-property relationships.

Machine learning driven performance for hole transport layer free
The rapid advancement of machine learning (ML) technology across diverse domains has provided a framework for discovering and rationalising materials and photovoltaic devices. This study

Machine learning-driven new material discovery
An overview of the application of machine learning in materials science. 12. 2. As an example, in the research of photovoltaic organic-inorganic hybrid perovskites by Shuai Hua Lu''s team, all the input data were obtained from reliable databases composed of high throughput first-principles calculations. The main goal of machine learning

(PDF) Machine learning in photovoltaic systems: A review
PDF | This paper presents a review of up-to-date Machine Learning (ML) techniques applied to photovoltaic (PV) systems, with a special focus on deep... | Find, read and cite all the research you

Application of machine learning in perovskite materials and
Machine learning potential (MLP), in which atomic coordinates, energies, and forces are collected to build a dataset, could greatly benefit from direct training models using data acquired from large databases, like MP. ML will be more widely used in materials science (e.g., photovoltaic materials). It is believed that ML will become an

6 FAQs about [Machine learning for material science photovoltaic]
Can machine learning be used in fabricating hole transport layer free carbon-based PSCs?
The rapid advancement of machine learning (ML) technology across diverse domains has provided a framework for discovering and rationalising materials and photovoltaic devices. This study introduces a five-step methodology for implementing ML models in fabricating hole transport layer (HTL) free carbon-based PSCs (C-PSC).
Can machine learning predict organic solar cells properties?
D. Padula, J. D. Simpson, A. Troisi, Combining electronic and structural features in machine learning models to predict organic solar cells properties. Mater. Horiz. 6, 343–349 (2019). E. O. Pyzer-Knapp, K. Li, A. Aspuru-Guzik, Learning from the harvard clean energy project: The use of neural networks to accelerate materials discovery. Adv. Funct.
Can deep learning predict organic photovoltaic materials?
Sun, W. et al. The use of deep learning to fast evaluate organic photovoltaic materials. Adv. Theory Simul. 2, 1800116 (2019). Padula, D., Simpson, J. D. & Troisi, A. Combining electronic and structural features in machine learning models to predict organic solar cells properties.
Can ml be used to design photovoltaic materials?
It is foreseeable that applying ML methods to the design of photovoltaic materials or systems will greatly accelerate the discovery of high-efficient materials, reduce the research lifecycle, and promote the development of OSCs.
Can machine learning be used in perovskite material design?
Application of machine learning in perovskite material design 3.1. Bandgap engineering in perovskites using ML
What is machine learning technology ml?
Machine learning technology ML can be classified into supervised learning, unsupervised learning, and reinforcement learning. Supervised learning is the most extensively used ML model as it can solve the regression and classification problems of various material systems .
Related Contents
- Top en 960 photovoltaic module testing machine
- Wholesale en 960 photovoltaic module testing machine
- Machine learning solar energy prediction
- Photovoltaic material meaning
- How do solar panels work photovoltaic cells live sciencelive science
- Does a cpap machine have a lithium battery
- Solar power for cpap machine
- Solar system learning resources
- Project based learning solar system
- Learning resources inflatable solar system
- Light energy storage material